· 4 min read
What is Forecasting Risk in Cybersecurity?
Learn about how to forecast risk in cybersecurity
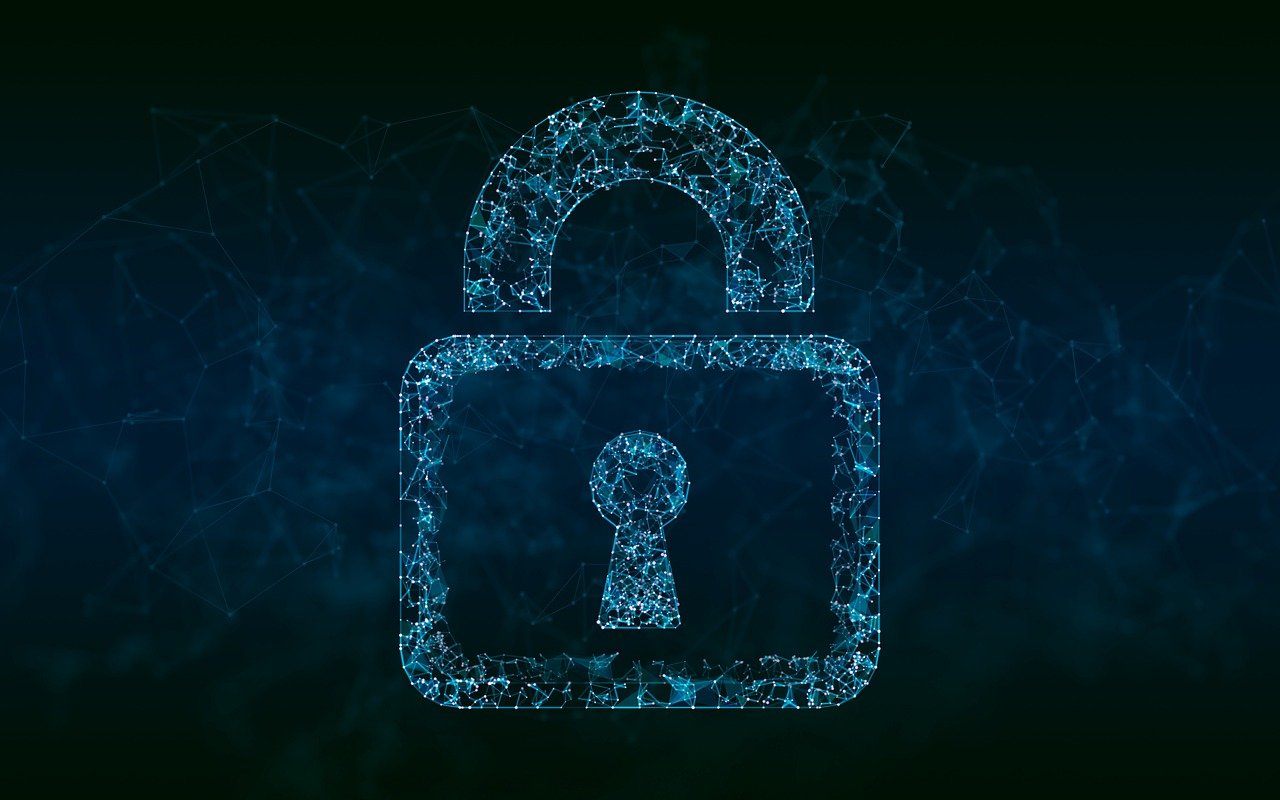
With cyber threats evolving in complexity and frequency, safeguarding data and systems is more critical than ever. A key component in strengthening cyber defenses is forecasting risk, a proactive approach to predicting and mitigating potential threats before they strike. But what exactly does forecasting risk in cybersecurity entail, and why is it so crucial?
Understanding Forecasting Risk
Forecasting risk in cybersecurity refers to the process of anticipating potential security threats and vulnerabilities that could affect an organization’s digital assets. It involves analyzing patterns, trends, and indicators from existing and emerging threats to predict future risks. This forward-thinking strategy allows organizations to implement preventive measures, allocate resources more effectively, and minimize potential damage.
Forecasting in cybersecurity is akin to weather forecasting—it doesn’t predict the exact threat but assesses probabilities, providing a strategic advantage in planning and risk management. By leveraging data analytics, threat intelligence, and historical attack patterns, forecasting can give organizations the insight needed to fortify their cyber defenses proactively.
Components of Cybersecurity Risk Forecasting
Effective forecasting in cybersecurity typically involves several key components:
1. Threat Intelligence Gathering
Collecting and analyzing data on current cyber threats is foundational. This can involve open-source intelligence, dark web monitoring, and sharing threat information through industry collaborations. Comprehensive threat intelligence provides valuable insights into the tactics, techniques, and procedures (TTPs) used by malicious actors.
2. Data Analysis and Machine Learning
Advanced data analytics and machine learning algorithms play a crucial role in making accurate predictions. By processing vast amounts of data, these technologies can detect anomalies, identify potential vulnerabilities, and recognize patterns that might indicate an impending cyber threat.
3. Trend Analysis
Understanding historical data and trends helps pinpoint common attack vectors and develop models to anticipate future threats. Trend analysis can aid in identifying shifts in the cybersecurity landscape and adapting strategies accordingly.
4. Scenario Planning and Simulation
Creating scenarios and running simulations helps organizations assess their preparedness for various types of cyberattacks. By simulating attacks, companies can evaluate their defenses, recognize weaknesses, and improve incident response plans.
5. Continuous Monitoring and Adaptation
Cyber threats are dynamic; therefore, continuous monitoring and adaptation are vital. Real-time monitoring facilitates the timely detection of threats, while adaptation ensures that predictive models evolve alongside emerging threats.
Challenges in Cybersecurity Risk Forecasting
While forecasting offers substantial benefits, it is not without challenges. These include:
Data Quality and Availability: Accurate forecasts rely on high-quality, comprehensive data. Inconsistent or insufficient data can lead to incorrect predictions.
Rapidly Evolving Threats: Cyber threats are constantly changing, with new and sophisticated attack methods emerging regularly. Keeping predictive models up-to-date can be challenging.
Human Factor: Effective forecasting also depends on skilled cybersecurity professionals capable of interpreting data and making informed decisions.
Over-reliance on Technology: While technology plays a pivotal role in forecasting, it should complement, not replace, strategic human oversight.
The Importance of Forecasting Risk in Cybersecurity
Incorporating forecasting into cybersecurity strategies provides numerous advantages:
Proactive Defense: Shifting from reactive to proactive defense allows organizations to preempt attacks and reduce their impact.
Efficient Resource Allocation: By identifying high-risk areas, resources can be allocated more effectively, ensuring that critical vulnerabilities are addressed.
Improved Incident Response: Early detection of potential threats enables faster, more decisive incident responses, minimizing damage and recovery time.
Enhanced Resilience: Organizations can build more robust security architectures by understanding and preparing for potential threats, aiding in long-term resilience.
Conclusion
As cyber threats continue to grow in scope and complexity, forecasting risk emerges as an indispensable tool in the cybersecurity arsenal. By leveraging data analytics, machine learning, and threat intelligence, organizations can transition from a reactive stance to a proactive approach, effectively safeguarding digital assets and maintaining the trust of stakeholders. In a world where the cybersecurity landscape is ever-evolving, embracing forecasting risk is not just beneficial, it is essential for a sustainable business model.